My Statement to the US Senate AI Insight Forum on Privacy and Liability
Yesterday, I had the honor of speaking in the US Senate AI Insight Forum on privacy and liability. While the meeting, moderated by Senators Schumer, Rounds, Heinrich, and Young, was conducted as an off-the-record discussion to educate policymakers and staffers, each of the expert panelists was given the opportunity to prepare a written statement to be disseminated on Leader Schumer’s website.
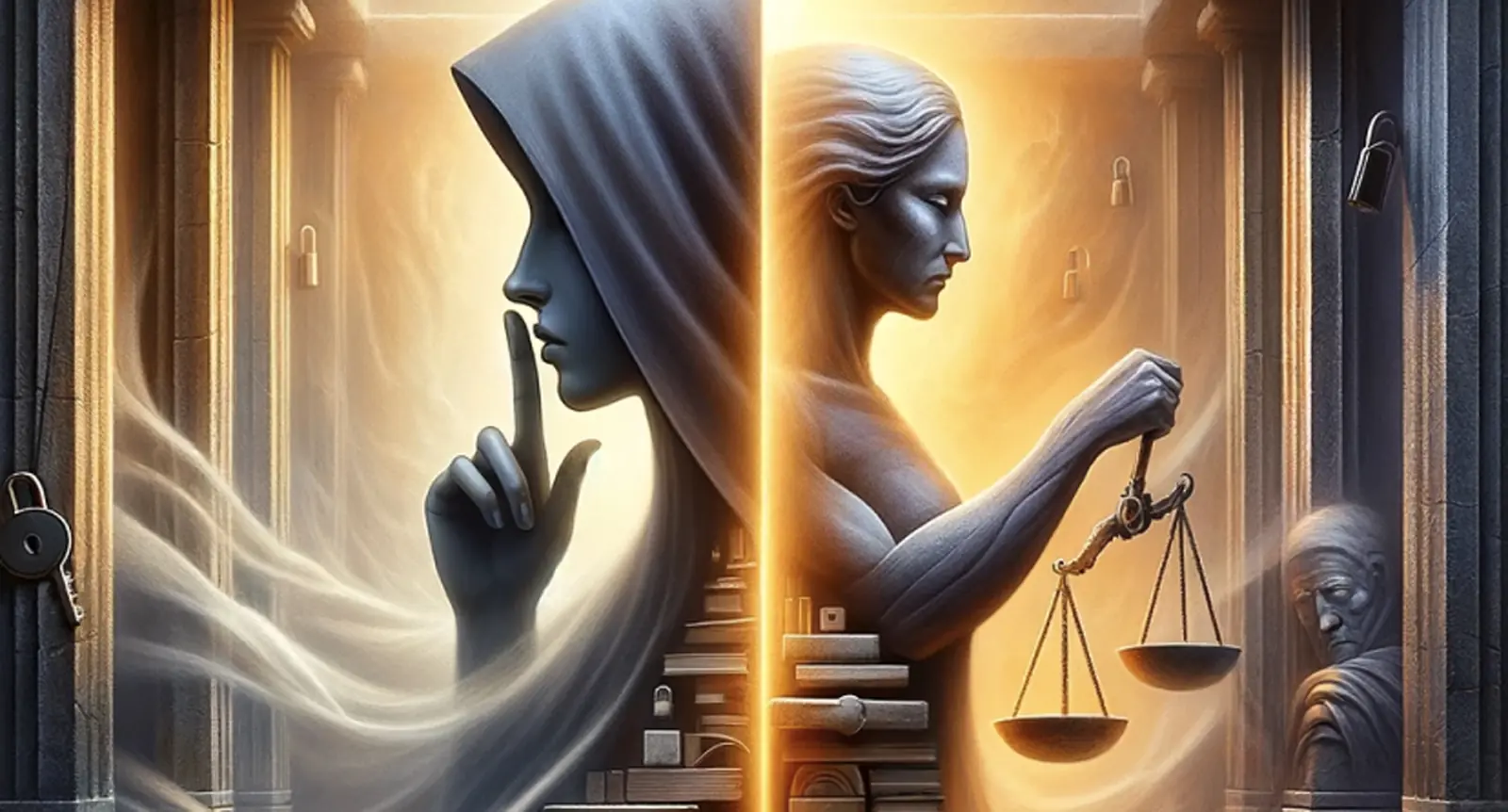
Yesterday, I had the honor of speaking in the US Senate AI Insight Forum on privacy and liability. While the meeting, moderated by Senators Schumer, Rounds, Heinrich, and Young, was conducted as an off-the-record discussion to educate policymakers and staffers, each of the expert panelists was given the opportunity to prepare a written statement to be disseminated on Leader Schumer’s website. Here’s what I had to share:
Focusing the AI Policy Conversation: From Calls to Action to Precise Guidance
Leader Schumer, Senator Rounds, Senator Heinrich, and Senator Young,
Thank you for this opportunity to speak with you at today’s AI Insight Forum about privacy and liability as they relate to AI policy, and to share this document which I have prepared in my personal capacity. I am an Assistant Professor of Machine Learning and Operations Research at Carnegie Mellon University, where my lab focuses on the responsible deployment of AI technology. Our priorities include the engineering foundations of building robust and adaptive models, bridging the gap between prediction and decision-making, and working across disciplinary boundaries to tackle the societal impacts and risks associated with the deployment of AI in consequential domains. I am also the Chief Scientific Officer for Abridge, a healthcare company whose Generative AI solutions transform raw audio of doctor-patient conversations into first drafts of clinical notes, combating the leading cause of physician burnout.
As a research scientist with subject matter expertise across the domains of trustworthy AI and as a technologist focused on healthcare innovation, I am aware of both the immense promise of AI-driven innovation for improving human life and also the risks associated with deploying this technology in consequential domains. In this statement, I will propose several key recommendations that I believe can guide policy-makers to support innovation while mitigating risks.
Avoid the Curse of Generality
What form should AI policy take? Should we aim for unified protocols that govern all activity? Or will every use case within every industry require a bespoke body of legislation? For better or worse, to effectively guide AI practitioners, policy must move in the direction of more focused, application-specific guidance.
Calls to action on AI, as captured in the recent Blueprint for an AI Bill of Rights and the recent Executive Order are serving a laudable role in spurring a national discussion on AI governance. How we should approach AI is characterized in broad terms, describing the generally desirable properties of such systems: safety, security, trustworthiness, unbiasedness.
Just like we cannot legislate human behavior to implore people to “be good”, progress towards actionable AI policy will require a narrowing of scope, which provides more specific guidance. Each specific AI application in each specific industry involves different potential harms, different levels of risk, different stakeholders with different degrees of agency, and different avenues for recourse. If we fail to scope AI policy appropriately, we risk either (i) devolving into mere platitudes; or (ii) imposing ill-conceived general requirements that may turn out to be wasteful or counterproductive in many settings.
Focus on the Right Details
To effectively guide practice, it’s not enough for AI policy to narrow the focus; that focus must target the right details. If we fail on this front, we risk instituting requirements that are toothless, brittle, or even harmful.
Consider, for example, that the recent Executive Order defines “foundation models” (general-purpose backbones trained on web scale data) in terms of the number of tunable parameters that they possess (here, 10s of billions). Unfortunately, this definition is extremely brittle. Despite years of research by machine learning theorists, our knowledge of just how the number of model parameters relates to model capabilities remains uncertain. Few researchers today would be shocked if a technical development next year led to equally performant models with 1/10th as many parameters. Given how fast the field is evolving, it would be wise to develop policy focused on the outcomes and attributes that really matter, rather than focusing on specific technical details of AI systems, like the number of parameters, or the kind of neural architecture employed.
Another concern would be tying policy requirements too closely to unproven technologies. For example, many regulatory proposals in the US and abroad have called to mandate the use of so-called “explainable AI methods”1, enumerating claims about how these methods can explain what’s going on inside the ‘AI black box’, can aid in debugging models, detecting unwanted biases, and can help practitioners to anticipate failures in robustness. To date, the literature does not substantiate these claims. The specific methods adopted by the explainable AI community (e.g., LIME, SHAP, and GradCAM) consist mostly of heuristics with only a dubious relationship to their claimed purposes2. Moreover, these methods generally all give conflicting answers3, and have failed most tests of efficacy when employed in human-in-the-loop experiments. The explainability narrative may sound good to the uninitiated, but falsely gives a sense of confidence and truth for AI technologies. In sum, AI policy should focus less on mandating specific categories of technical methods, and more on ensuring desired outcomes.
Finally, we should beware of anchoring policy to practices that lack clear definitions. For example, “red teaming” has recently emerged as a focal point of both corporate messaging and policy proposals around responsible AI. In AI “red teaming”, a group of researchers aims to stress test a model, exposing weaknesses or eliciting undesirable behaviors. Red teaming connotes taking a rigorous attitude towards one’s product, thinking creatively and acting persistently to find its weaknesses. It’s a good practice and a good attitude to have. However, what precise activities constitute red teaming remains frustratingly vague; it’s more a “vibe” than a concrete practice. Effective policy should center around well-defined practices.
Liability Should be Guided by Considerations of Human Agency
Consider two scenarios involving an application of a chatbot to medical diagnostics.
Scenario One (autonomous chatbot): Consider a situation, however unrealistic, in which an AI-powered chatbot was applied to make medical diagnoses and issue treatment plans. Suppose that without any human physician in the loop, the chatbot directly interacted with a patient, ultimately outputting a diagnosis and treatment plan, which were automatically entered into the patient’s record and acted upon. Here, the behavior of the AI system, and thus the actions of the AI developer directly influence patient care, with no opportunity or expectation for physician intervention or supervision.
Scenario Two (assistive chatbot): An AI-powered chatbot is accessed by a physician to aid in developing a differential diagnosis, or list of potential diagnoses to explain the patient’s ailments. The AI system might return incorrect information, poor suggestions, or even confabulate references to medical research that doesn’t exist. The physician interprets the chatbot output and ultimately makes the final decision on a diagnosis and treatment plan.
These two examples highlight the challenges in using a rigid ‘one-size-fits-all’ approach to liability. While we may still debate precisely how to think about liability in each of these scenarios, it seems clear that they are fundamentally different in a profound way. In scenario one, all liability must rest with those developing and deploying the technology. In scenario two, the system still ingests information about a patient and outputs possible diagnoses and treatment protocols. However, without the ability to directly influence patient care, it becomes hard to identify what fundamentally differentiates this usage pattern from a doctor using Google search. Indeed, doctors frequently search the web, and frequently encounter inaccurate or unhelpful content. And yet Google is seldom held responsible for medical malpractice. Doctors are entrusted to search the web in the course of administering care because we trust their ability to calibrate their trust levels, evaluate the reliability of sources, and fact-check any information acquired. While there is a healthy debate to be had over what precise regulations should apply at each point along the autonomy spectrum, it is clear that the level of agency retained by the human decision-maker is a critical factor that must influence these determinations.
Focus AI Policy on Novel Aspects—Example: Privacy in Medical Documentation
Because my private sector work as Chief Scientific Officer of Abridge sits at the intersection of AI and healthcare, I am frequently asked how I think about the interplay between privacy and AI. Sometimes people are surprised to find out that my biggest concerns regarding privacy, by far, are those that command the attention of any responsible technologist operating in the healthcare industry today: aspiring towards the highest standards for cybersecurity, including ensuring the secure transmission and storage of all data; maintaining strict access controls; and faithfully upholding all regulatory and contractual requirements around data handling and retention. Notably, these challenges are not new; they are faced by most enterprise partners in the healthcare space, including electronic medical records, insurers, and scribing companies. While the application of AI methods in our domain does indeed introduce novel concerns, it’s important to situate these against the backdrop of the more formidable privacy challenges that our enterprises already face every day.
So what precisely changes vis-a-vis privacy? One longstanding concern among machine learning research scientists is that AI models trained on sensitive data might overfit to this data, effectively “memorizing” these examples. As a result, the AI could potentially regurgitate sensitive information about one patient when generating output that could be visible to other patients or clinicians. From a scientific standpoint, such cases of verbatim regurgitation are theoretically plausible even if cases of privacy leakage via model regurgitation have been somewhat rare in practice. In principle, we expect this risk to be greater when models are trained for long amounts of time on smaller datasets. Conversely, the risks become less pronounced when models are trained on massive datasets, revisiting each data point fewer times.
Fortunately, there are at least two natural remedies that can mitigate these concerns. First, we can conduct robust evaluations of our models on large quantities of inputs to quantify how often, if ever, the models regurgitate sensitive content from the training data. A second, more robust solution that many of us already undertake is to train our models only on de-identified patient data, substantially reducing the novel privacy risks. While some parties might see the broader picture of (i) new companies; (ii) lots of data; and (iii) using data in novel ways, and call for a broader rethinking of data collection, use and retention policy, it is important to distinguish between the status quo problems faced by most existing companies and anticipated by existing legislation, versus those novel privacy problems that arise due to the introduction of AI systems.
Conclusion:
Recent calls to action on AI regulation represent a laudable first step towards crafting policy frameworks that could support innovation while ensuring the responsible deployment of AI systems. However, advancing to the level of concrete policy recommendations will require crafting industry- and application-specific guidance. Crucially, AI policy around liability and privacy should focus not only on the capabilities of the underlying AI technology but also the broader software system and business process in which it is deployed, accounting thoughtfully for how the level of human agency influences the risk landscape. Moreover, our first efforts at AI policy should concentrate precisely on novel risks due to the introduction of this technology, rather than familiar problems with established norms and protocols.
Want to learn more about how Abridge can help?
Contact us.png)
Zachary Lipton, PhD
Zachary Lipton is the Chief Technologist and Scientific Officer of Abridge and a Professor on the Machine Learning faculty at Carnegie Mellon University. His research focuses include the theoretical and engineering foundations of robust and adaptive machine learning algorithms, applications to both prediction and decision-making problems in clinical medicine, natural language processing, and the impact of machine learning systems on society.